Advancing the understanding and management of Neofabraea spp. in apples: Inoculation protocols, spore monitoring & crop protection strategies in organic farming
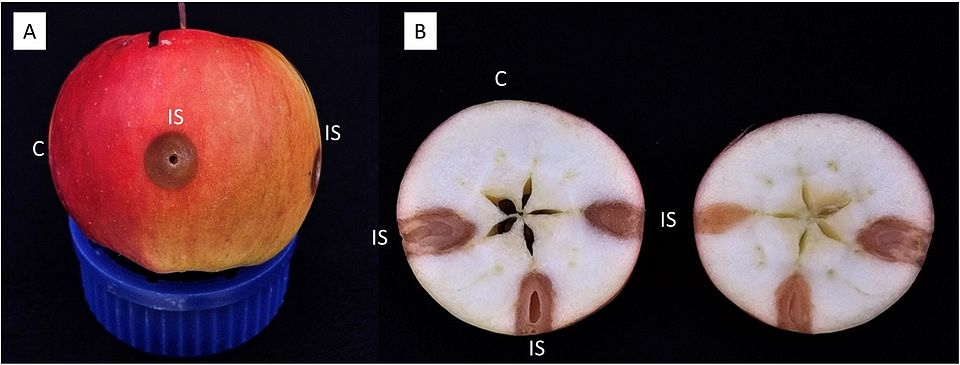
MScAndreas Berlepsch-Valendas
02/2025
Supervision: Dr. Pascale Flury (University of Basel) and Dr. Alessio Bernasconi (FiBL)
Abstract:
Apples rank among the most popular fruits in Switzerland and are generating the highest revenue in the fruit market. During the storage of apples, major losses are attributed to the pathogen Neofabraea spp. Despite the fact that different species of this genus are the main cause of postharvest losses in apples, little is known about the biology of this pathogen. The objective of this study was to address the existing knowledge gaps by conducting experiments that target multiple aspects of the pathogen's biology and additionally explore diverse management- and monitoring strategies. Three main research directions were approached in this study: (1) development of an inoculation protocol to study N. alba infections in apples, (2) establishment of methods for monitoring spore dispersal and infection timepoints, and (3) storage trials, where strategies and management strategies in organic farming against the pathogen were tested. A reliable inoculation protocol with a high success rate was developed to test the efficacy of different plant protection products. The establishment of a qPCR assay to monitor Neofabraea spores in the field revealed the presence of spores during the whole sampling period (June till October). The storage trials highlighted the critical role of proper storage conditions in managing postharvest apple quality; however, none of the tested products demonstrated efficacy against Neofabraea spp. The results provide a basis for up-scaled monitoring experiments of the pathogen as well as the testing of plant protection products. Furthermore, this work highlights key aspects of the biology of Neofabraea and offers insights for its in vitro handling.
In a fast-changing climate, the need for more sustainable agricultural practices is unprecedented. Field inoculation with arbuscular mycorrhizal fungi is a promising alternative to mineral fertilizers and pesticides but this method suffers from a high context-dependency. Models using soil physiochemical parameters and fungal communities aimed to solve this problem but never considered the contribution of soil bacteria. Additionally, microbiome sequencing is costly and threatens the democratization of such models with farmers. With this thesis we aimed to investigate the potential of Random Forest in fungal microbiome predictions and assessed the contribution of bacteria to the predictive model of mycorrhizal growth response.
We used data produced in a large-scale field inoculation experiment to compute a finely tuned Random Forest model and used leave-one-out validation to assess the accuracy of our approach. Additionally, we performed 16S full-length soil profiling and used three different methods to find soil bacteria responsible of context-dependency and produced models made of soil parameters, fungal and bacterial communities. Finally, we conducted ten field inoculation experiments to validate the previous models in an uncovered geographic area.
Random Forest model gave decent performance in most of the fields and revealed non-linear patterns shaping soil fungal communities. 13 soil bacteria were found to be indicative of high or low mycorrhizal growth response and their addition to the models increased the covered variance up to 95 %. Moreover, cross validation of model that could recommend inoculation to farmer resulted in an accuracy of 96 %. Nevertheless, the model validation on new fields resulted in inaccurate predictions because of model overfitting.
In this work we showed that microbial inoculation would benefit from such models to reduce the costs of sequencing and increase inoculation success.